Generative AI in Embedded Finance
AI Is Coming to Embedded Finance, Here’s What Fintechs and Brands Should Know
7 Min Read

Eric Rhea
Principal Engineer, Helix
ChatGPT on your mind? It’s now impossible to ignore, and it’s why AI is coming to embedded finance.
As the media-charged ChatGPT buzz heightens, so does industry interest in large language models (LLMs), generative-pretrained transformers (GPTs), and the innovation across the open-source community. It’s a good time to stop and consider the significance and opportunity today’s artificial intelligence (AI) presents—from your front-row seat to history.
Let’s break it all down to understand:
- What the generative AI revolution—and specifically ChatGPT—means for financial services
- The considerations for brands and fintechs before they jump in
- Real-world AI applications for finance and banking
Putting generative AI in perspective
As ChatGPT has its moment, we are witnessing the most significant cognitive revolution since Gutenberg’s printing press.
In or around 1455, goldsmith Johannes Gutenberg invented the first transformative cognitive technology. For centuries prior, book printing was a highly labor-intensive and time-consuming endeavor, with each book made individually.
The invention of the printing press—its movable blocks, paper, and ink—made it possible to produce books quickly and far less expensively than before. It meant information could be widely distributed for the first time. It replaced scribe with machine and changed the world forever.
The printing press powered wide dissemination of not just books, but also financial records, contracts, and promissory notes. It laid the foundation for the standardization and widespread use of documents that eventually evolved into the modern financial systems we know today.
Centuries after the printing press revolution, we are now witness to the similarly revolutionary generative AI. This new cognitive technology again accelerates the spread of information in once-unimaginable ways, fueling giant leaps in both human connection and operational efficiency. Generative AI is a metaphorical new printing press, and it can help make finance human.
Why AI matters in embedded finance
The power of embedded finance is in its ability to uniquely solve real problems for people through personalized banking experiences. The cognitive revolution of AI supercharges personalization and those experiences.
Imagine technology that can quickly understand context to craft personalized experiences. It will shift embedded finance product visions, and the products ultimately delivered. Until this rev of AI, machines could not produce output indistinguishable from humans. But now, new generative AI models can engage in sophisticated, human-like conversations with users and quickly generate seemingly original content.
“This is a boon for personalized experiences.”
LLM draws on the corpus of all content it’s trained on through every interaction. These vast datasets enable LLM systems to link concepts that drive compelling results. The outputs could include fixing broken code, writing marketing plans, or even helping take products to market.
In the last two months, ChatGPT grew from zero to 100 million users. Use cases are growing and crystalizing. The technology is accelerating on all fronts, and ChatGPT is not the only solution of its kind. This is only the beginning.
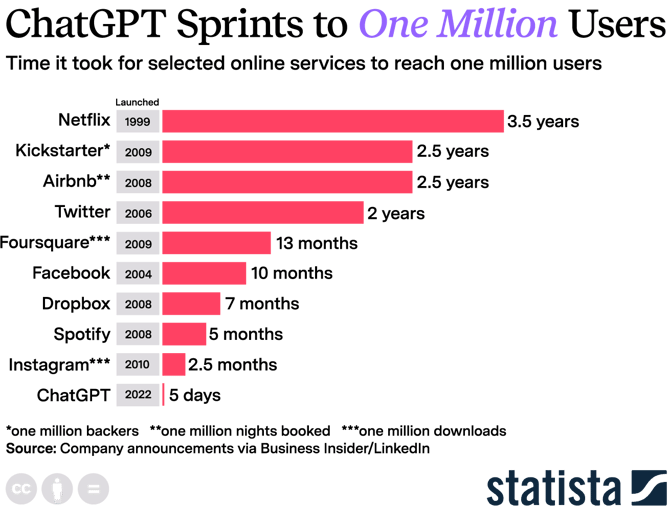
While AI is not new to banking and finance—think high-frequency trading, fraud detection systems, identity scanning, and expert rules-based systems—what’s happening now and coming from this amped-up technology is completely next-level.
A significant strength of LLM is how it functions. It works through a prompt, a simple query you ask an AI text assistant. The assistant then answers using its vast understanding of language and context to provide useful information or insights. With the right prompt, LLM can become anyone, from chef to Wharton MBA graduate, even life coach. The open-source community maintains an impressive collection of prompts within GitHub.
The possibilities to create personalized experiences to solve very targeted, truly life-enhancing problems, for all kinds of people, is extraordinary.
What are the practical applications in financial services?
Deeper customer connections
According to Gartner, only 15 percent of customer interactions create meaningful value enhancement. AI-enabled personalization can increase meaningful connections that deliver more value to customers and drive long-term loyalty. That loyalty drives down costs and hastens the charge toward profitability.
Here are examples of familiar financial services brands, early adopters of GPT-4, putting AI to work for better customer experiences and deeper connections:
Stripe
With OpenAI’s LLM, GPT-3 Stripe improved user experience in its support ticket routing and question summarization, resulting in better customer service. Going further, earlier this year, the company challenged 100 of its employees to dream up value-add features and functionality using the new GPT-4. From 50 submissions, it explored 15 that delivered increased value through personalization. Ultimately, it found practical applications that generated even more measurable results in the areas of customer support, as well as cost-savings through fraud-detection improvements.
Morgan Stanley
Morgan Stanley is using GPT-4 to power an internal chatbot that searches its enormous, unwieldy library of investment strategies, market research, and analyst insights across numerous internal sites, mostly in PDF format. Now, with GPT-4, financial advisors ask questions, and a bot quickly delivers answers in an easily digestible format. For Morgan Stanley customers, the results mean more relevant and effective, personalized financial solutions.
Gartner research supports that personalized, higher-value interactions keep customers and increase their lifetime value. Imagine the effects of near-instant access—through a conversational chat interface —to any information, anywhere in your organization and even far beyond.
Operational efficiencies, cost savings
GitHub and Helix
When GitHub launched Co-Pilot in 2021, it was billed the AI Pair Programmer, promising to reduce redundant tasks for technologists at all levels. For our team at Helix, the idea of improving coding time by 55 percent was more than we could resist.
It meant one person could instantly become many and drive major efficiency. We tested it and found GitHub’s published numbers impressively accurate. So much so, my engineers have asked, “How does it know what I’m thinking?”
For fintechs and brands pursuing embedded finance for profitable growth, more-cost-efficient operations are a big part of getting there. Code completion AI tools—GitHub is just one—can be a productivity boost with material effects
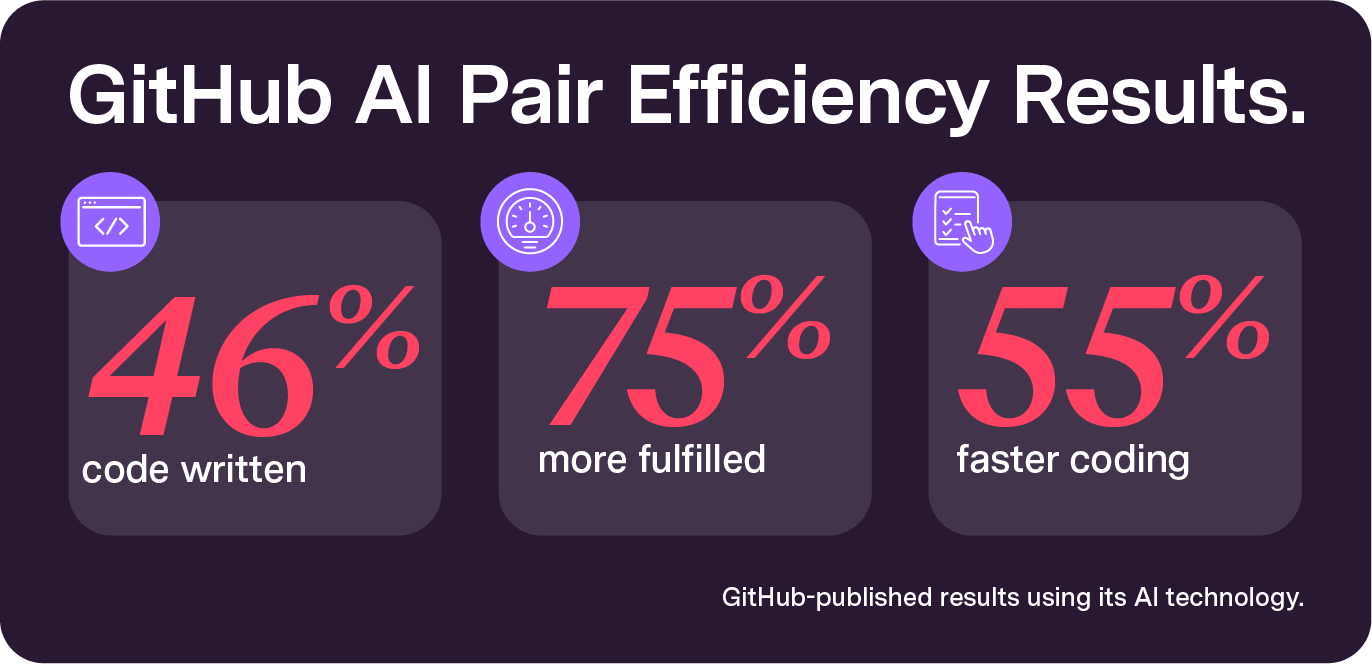
And this isn’t an efficiency win only for your software engineering teams. LLM delivers optimization benefits that spill out from engineering into your product organization and its ability to deliver products to market faster.
There are important considerations too
Before jumping in completely—or even getting your feet wet—with generative AI, there are key considerations to explore related to potential legal, ethical, and economic implications. Start with these:
The fine print
Like any new technology, there’s a good amount of fine print to consider with AI. In fact, ensure your risk review, legal, and compliance teams are studying the terms of service for public AI solutions like ChatGPT, which use public domain inputs to train.
Enhancing, not replacing people
With generative AI, computers can exhibit the “creative” thinking to produce original content to respond to questions, based on huge volumes of collected data and interactions. They can write your blog, sketch your logo, respond to customers, and write your computer code.
You may have even experienced how convincing these systems can be at expressing their points of view. They’ll print anything you ask for, and sometimes even content you didn’t. A model can go too far. In one example, it almost-convincingly argued that, based on the data, water isn’t wet.
Historically, GPTs and LLMs have been trained on publicly available content, including everything from articles and books to Reddit discussions, social media content, and Wikipedia. As a result, the data is exposed to bias, discrimination, and inaccuracy.
All this can become a real risk to production-level deployments, but this can be managed with a human-in-the-loop component to guide accurate output. Human expertise is required to ensure more factual responses. Human-in-the-loop, combined with AI trained on exclusively internal data, may be the best option in some applications.
Privacy and confidentiality
Without proper risk management and due diligence, employees can unintentionally share business secrets with generative AI. That’s, again, because in many of the freely available systems, inputs are deliberately captured for machine learning and to train future releases of the technology. Another inherent danger in this process is that, if trained on your inputs, your trade secrets can be broadcast to the world. Large enterprises such as Amazon have even issued warnings about this.
But much of the risk can be potentially managed without complex engineering efforts, including through effective contractual agreements. An agreement of terms regarding your data is not a new idea. Ensure that when engaging with vendors or platforms providing these key capabilities that you have a clear understanding of the relationship of model training to the inputs you are providing to those systems.
There may be systems where you’re quite comfortable with the inherent model improvements, such as with vendors that have specialized fraud solutions. But balancing that risk is part of doing business, and it’s critical to manage it wisely.
Exploring possibility
AI systems can be deployed within your organizations right now to do remarkable things, especially combined with the right partners, processes and supporting technology. While there’s still much more to come, and much to explore, learn and consider, generative AI will play an important part in embedded finance and making finance human.
Helix experts work with fintechs and brands to bring ideas to life and drive profitable growth. If you’d like to start exploring with our team of experts, we’re ready to start the conversation with you.
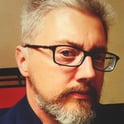
Eric Rhea
Eric Rhea is principal engineer at Helix by Q2.
Eric writes here from a perspective informed by his experiences as:
- An original engineer behind CorePro, which was one of the first BaaS platforms and became Helix
- A passionate technology explorer and creator
- Thought leader and speaker on embedded finance technology and the application of artificial intelligence to solve problems